Deepfake technology creates highly realistic media by manipulating video, audio, and images. This technology has blurred the line between reality and fabrication, posing significant threats across industries. From phishing attacks and misinformation campaigns to identity theft, deepfakes undermine security, trust, and privacy on a global scale.
As deepfakes grow more sophisticated, detecting them has become essential to maintaining the integrity of digital media. Reliable detection methods are critical for safeguarding cybersecurity, preventing fraud, and combating misinformation. Advanced deepfake detection software addresses these challenges, offering vital tools to identify and mitigate the risks of manipulated content.
What Are Deepfakes?
Deepfakes are created using artificial intelligence, most commonly Generative Adversarial Networks (GANs), to generate highly realistic synthetic media. GANs use two neural networks working in tandem: the generator creates synthetic content, while the discriminator evaluates its authenticity. Through repeated iterations, the generator refines its output until it is nearly indistinguishable from genuine media.
These technologies have both positive and negative applications. In entertainment, they enable realistic CGI effects and the recreation of historical figures. Educationally, they power simulations and accessibility tools, such as language translation and voiceovers. However, deepfakes have also been weaponized for impersonation scams, phishing attacks, and misinformation campaigns, eroding public trust and creating security vulnerabilities. On a personal level, they have facilitated privacy invasions, including identity theft and the distribution of non-consensual synthetic media.
Detecting deepfakes is challenging due to their ability to replicate natural features like lighting, facial expressions, and speech patterns with precision. Moreover, their adaptive algorithms continuously evolve, bypassing traditional detection methods. With tools to create deepfakes becoming widely accessible, manual detection is no longer sufficient. Advanced detection technologies are vital to address this growing threat effectively.
The Core Technologies Behind Deepfake Detection
AI and Machine Learning in Detection
Deepfake detection relies heavily on artificial intelligence and machine learning to analyze and identify anomalies in media that may indicate manipulation. AI systems are trained using large datasets of real and synthetic media, enabling them to recognize patterns and inconsistencies that are not immediately apparent to the human eye. Machine learning algorithms, particularly deep learning, excel at processing complex datasets and uncovering subtle discrepancies in audio, video, and image files.
These technologies empower detection tools to not only identify manipulations in static images but also analyze dynamic elements such as motion, facial expressions, and voice patterns in videos. The adaptability of machine learning models allows them to improve over time as they are exposed to more examples of both authentic and manipulated content. This constant evolution is critical in staying ahead of increasingly sophisticated deepfake generation methods.
Role of Neural Networks in Analyzing Media
Neural networks play a central role in deepfake detection by mimicking the way the human brain processes information. Convolutional Neural Networks (CNNs) are particularly effective for analyzing visual data, as they can identify intricate pixel-level inconsistencies in images or videos. For instance, CNNs may detect subtle differences in lighting, shadows, or edge artifacts that suggest tampering.
Recurrent Neural Networks (RNNs) and Long Short-Term Memory networks (LSTMs) are often employed for analyzing sequential data, such as audio or video frames. These models excel at tracking temporal patterns, enabling them to identify inconsistencies in speech, lip-syncing, or movement across frames. Neural networks work in tandem to process various layers of information, providing a comprehensive analysis of media for deepfake detection.
Key Methods
Deepfake detection employs a variety of methods, each targeting specific aspects of manipulated media. Forensic analysis focuses on identifying anomalies in the physical characteristics of media. It also examines biological inconsistencies, including unnatural blink rates, facial asymmetry, or abrupt movements that are uncharacteristic of human behavior.
Metadata inspection plays a complementary role by analyzing the hidden data within media files. This includes examining timestamps, compression artifacts, or inconsistencies in encoding that may suggest tampering. While metadata alone may not always confirm a deepfake, it can provide crucial context for further analysis.
GAN differentiation is another critical method, leveraging the very technology used to create deepfakes to detect them. By understanding how Generative Adversarial Networks function, detection systems can identify telltale signs left behind by the generation process, such as repetitive patterns, noise, or overfitting artifacts. Advanced detection models are trained specifically to spot these markers, even as deepfake generators become more sophisticated.
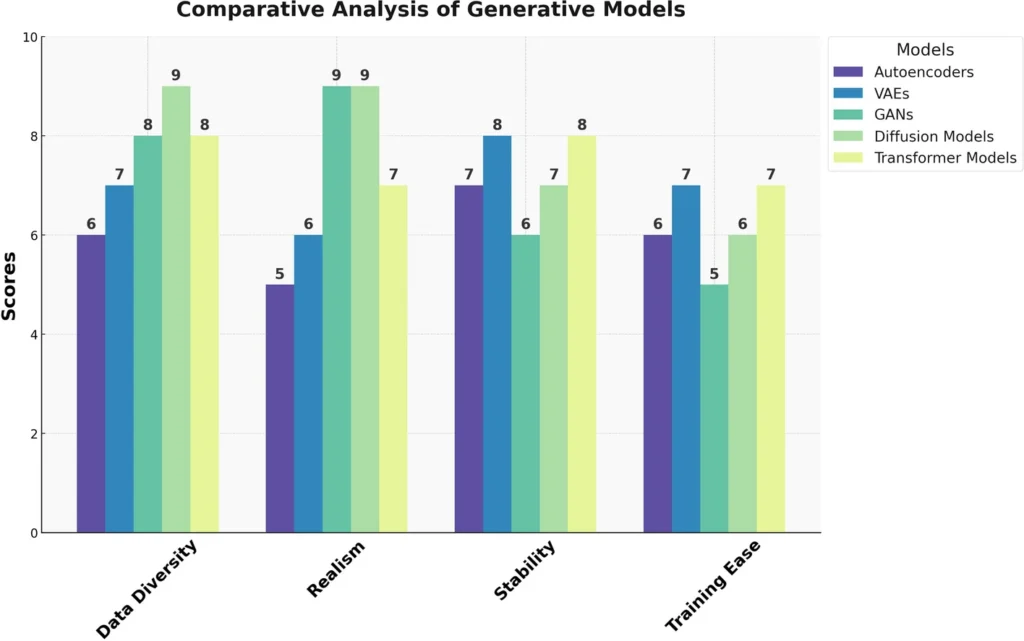
Key Features of Effective Deepfake Detection Software
Effective deepfake detection software is built on a foundation of advanced analytical capabilities designed to uncover manipulations in media. These systems employ cutting-edge technologies to examine visual, audio, and behavioral cues that are often imperceptible to the human eye or ear. Here are the key features that make deepfake detection software robust and reliable.
Analysis of Visual Cues
One of the primary methods for detecting deepfakes lies in scrutinizing visual elements of media. Deepfake algorithms, while sophisticated, often leave behind subtle inconsistencies in areas such as reflections, and textures. For example, shadows may appear unnaturally aligned, or lighting gradients may fail to match the environment. Additionally, deepfake models sometimes generate artifacts or irregularities at the pixel level, particularly around the edges of facial features like eyes, lips, or hair.
Advanced detection tools utilize Convolutional Neural Networks (CNNs) to process images and videos frame by frame, identifying these anomalies with precision. By comparing visual data against patterns observed in genuine media, the software can flag manipulated content with a high degree of accuracy.
Facial recognition market size worldwide in 2020 and 2025 (in billion U.S. dollars)
Audio and Voice Recognition Patterns
Deepfake detection software also incorporates audio analysis to identify discrepancies in synthetic speech. AI-generated voices often lack the nuanced intonation, pitch variation, and rhythm found in natural human speech. Sophisticated tools analyze voice frequencies and speech patterns to detect signs of artificial manipulation, such as abrupt transitions, robotic undertones, or missing emotional inflections.
In cases where deepfakes combine mismatched video and audio (e.g., lip-sync deepfakes), detection algorithms cross-check audio cues with facial movements. These tools can identify mismatches between spoken words and corresponding lip movements, exposing the manipulation.
Behavioral Pattern Identification
Human behavior, particularly in facial expressions and movements, follows consistent patterns that deepfake algorithms struggle to replicate authentically. Effective deepfake detection software examines these behavioral markers for irregularities. For instance, unnatural facial expressions, inconsistent blinking rates, or mechanical head movements can indicate synthetic content.
Tools equipped with motion analysis algorithms monitor the fluidity and realism of movements within video frames. For example, deepfakes often fail to accurately render subtle microexpressions or natural transitions between emotions. By comparing these behaviors to data from authentic recordings, the software can pinpoint discrepancies indicative of tampering.
These features—visual analysis, audio scrutiny, and behavioral monitoring—work together to create a comprehensive detection framework. The result is a powerful tool capable of identifying even the most subtle manipulations in multimedia content, ensuring authenticity and reliability in an era of increasingly sophisticated deepfake technology.
The deepfake computer based intelligence market size has filled dramatically lately. It will develop from $0.65 billion of every 2023 to $0.82 billion out of 2024 at a build yearly development rate (CAGR) of 25.9%. |
Process of Deepfake Detection
The process of deepfake detection involves several stages, each employing advanced technologies to analyze and identify manipulated content. From ingesting media to implementing real-time detection, this systematic approach ensures a thorough examination of potential deepfakes.
Ingestion and Preprocessing of Media
The first step in deepfake detection involves the ingestion and preprocessing of media, encompassing video, images, or audio. During ingestion, raw data is collected from various sources, such as file uploads, live streams, or online content. Preprocessing follows to standardize the media, ensuring consistency and compatibility for analysis. This stage includes resizing and normalizing the resolution and scale of media, converting files into formats suitable for streamlined processing, and reducing noise by removing artifacts or compression distortions that could interfere with detection. By refining the input data, preprocessing sets the foundation for accurate and efficient feature extraction and analysis in subsequent stages.
Feature Extraction and Model Training for Detection
Once the media is prepared, the detection system extracts key features to analyze. For images and videos, this involves examining pixel-level details, facial landmarks, and motion patterns. For audio, it includes analyzing waveforms, pitch, and speech cadence. Feature extraction helps the software identify anomalies that are common in deepfakes, inconsistencies in speech, or irregular motion.
The system is powered by AI models trained on extensive datasets of real and fake media. During the training phase, these models learn to recognize distinguishing characteristics of authentic content and the artifacts introduced by deepfake algorithms. Generative Adversarial Networks (GANs) are often used in training to create synthetic examples, enabling the detection models to adapt to evolving deepfake techniques.
Use of Datasets for Detecting Manipulations
Datasets play a critical role in training and validating deepfake detection systems. These datasets include thousands of examples of both real and manipulated media, providing a foundation for machine learning models to identify patterns and anomalies. Popular datasets such as FaceForensics++ and DFDC (Deepfake Detection Challenge) are commonly used in this domain.
High-quality datasets are diverse, covering various demographic, environmental, and contextual factors. This diversity helps detection models generalize across different types of media and adapt to the latest deepfake generation techniques. The models continuously improve as they are exposed to updated datasets, ensuring they remain effective against emerging threats.
Real-Time Detection Algorithms and Automation
Modern deepfake detection systems integrate real-time algorithms and automation to address the rapid generation and dissemination of manipulated content. These systems are designed to analyze streaming media or process large datasets without requiring manual intervention. Real-time algorithms operate by examining video frames or audio segments as they are received, applying threshold-based detection to identify and flag content with a high likelihood of manipulation. The results are delivered almost instantly, enabling swift action against identified deepfakes. Automation further enhances scalability, allowing these systems to manage vast amounts of data efficiently. This capability is critical for applications like content moderation, cybersecurity, and live event monitoring, ensuring that deepfakes are detected and mitigated as quickly as they emerge.
Applications of Deepfake Detection Software
Deepfake detection software is essential across various industries where the authenticity of media is crucial. From protecting sensitive information to upholding public trust, its applications are widespread and growing as deepfake technology evolves.
Use Cases in Law Enforcement and Cybersecurity
Law enforcement agencies rely on deepfake detection tools to combat identity fraud, impersonation scams, and the spread of manipulated evidence. Deepfakes have been used in phishing schemes, creating false narratives or videos that impersonate high-profile figures to manipulate targets. By identifying fake content early, detection software helps safeguard individuals and organizations from these malicious activities. In cybersecurity, the technology is critical for preventing breaches caused by deepfake-generated voice commands or fake video authentication systems. It is a vital defense in protecting sensitive systems and preventing AI-driven attacks.
Applications in Media Verification and Journalism
Deepfake detection is increasingly used in media and journalism to verify the authenticity of news content. With manipulated videos and fabricated speeches becoming tools for misinformation campaigns, ensuring the credibility of news sources has become a priority. Journalists and fact-checkers use detection software to analyze video and audio evidence, flagging potential deepfakes before they reach the public. This technology not only prevents the spread of misinformation but also helps restore public trust in media outlets and the content they distribute.
Corporate Security and Fraud Prevention
Corporations utilize deepfake detection tools to protect their operations from fraud and reputational damage. Impersonation attacks, where fake videos or audio clips of executives are used to authorize transactions or spread false information, pose a significant threat to businesses. Detection software mitigates these risks by identifying manipulated media and preventing fraudulent activities. Additionally, organizations use these tools to safeguard their brands by detecting and countering fake advertisements or media that could harm their public image.
How Many Businesses Are Affected by Deepfake Fraud?
Future Trends in Deepfake Detection
The future of deepfake detection will be shaped by advancements in artificial intelligence, cross-sector collaboration, and the need to address emerging challenges as the technology becomes more accessible. AI innovations, such as self-supervised learning and explainable AI, will enhance the ability of detection systems to identify subtle manipulations with greater accuracy and adaptability, even as generative models evolve. Collaboration between academia, industry, and governments will be crucial, with academic institutions driving research, industries scaling applications, and governments implementing regulations and funding initiatives. However, the increasing accessibility of deepfake creation tools, enabled by open-source software and user-friendly interfaces, poses significant challenges. Detection systems must not only adapt to a wider range of manipulations but also navigate ethical concerns around privacy and misuse of detection technology. These combined efforts will ensure that deepfake detection keeps pace with evolving threats, safeguarding the integrity of digital media.